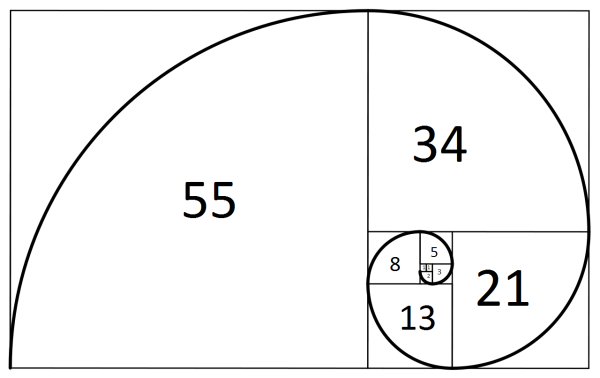
Default Argument Tricks
813 words. Time to Read: About 8 minutes.The other day I was working on a code challenge on CodeWars. I finished it up, feeling proud of myself (as one does), and moved on with my day. A little while later, it struck me that I had done something that few of the other people that completed the challenge had done, and my solution (I thought humbly) was more elegant than some. Here is a paraphrasing of the challenge.
The following code snippet is a python version of an algorithm to produce the n-th number of the Fibonacci sequence. For those that don’t know, the Fibonacci sequence is a sequence of numbers beginning with 0 and 1. After that, each number, n, is calculated: n = (n-1) + (n-2). More simply, each number is the sum to the two preceding numbers. 0, 1, 1, 2, 3, 5, 8, 13, 21… The Fibonacci sequence is a good way to introduce recursive tree algorithms. Without further ado, here’s a version of the algorithm.
def fibonacci(n):
"""Takes integer n. Returns n-th Fibonacci number (integer)."""
if n in [0, 1]:
return n
return fibonacci(n - 1) + fibonacci(n - 2)
Note that this algorithm is prohibitively inefficient. It double calculates each number. This solution effectively doesn’t work for n > 50 or so. One way to solve this is “memoization”, or caching the calculated results to speed up the process. Create a memoized fibonacci function that keeps track of its calculated values. Bonus points for making this cache private to the function.
I highly encourage you to give this a try on your own. There are lots of entirely valid ways. The following is a SPOILER, and it gets the job done with a private cache. Most importantly, it capitalizes on a feature of the language that is generally a common trip-up point for those newer to the concept. Check it out!
def memoFib(n, cache=[0, 1]):
"""Takes integer n and optional preloaded list of integers, cache.
Returns the n-th Fibonacci number, and stores the results for future calculations."""
if n < len(cache):
return cache[n]
else:
result = memoFib(n - 1) + memoFib(n - 2)
cache.append(result)
return result
If you see what I did, congratulations! You’re amazing! If not, congratulations! You are about to be amazing! Here’s the trick: in python (and other languages), if you have a default parameter in a function that is a mutable value, python doesn’t create a new one for each function call, it uses the same one every time. I’ll explain more clearly. Try the following.
def function_with_immutable_default(a, b=3):
"""3 is an immutable number. If you fiddle with b, it will
simply make a copy because you can't, by definition, change/mutate it"""
if a > 0:
b = 5
return b
$ function_with_immutable_default(1)
5
$ function_with_immutable_default(-1)
3
def function_with_mutable_default(a, b=[]):
"""Mutables are anything that can be modified in place without
creating a new copy."""
if a > 0:
b.append(5)
return b
$ function_with_mutable_default(1)
[5]
$ function_with_mutable_default(-1)
[5]
$ function_with_mutable_default(2)
[5, 5]
Do you begin to see? In the second example, the list that is getting assigned to b doesn’t stand for a theoretical list that will get created every time the function is called. You are assigning that particular list to b. Anytime the function gets called, it will reach for that list. If you call the function 5 times, that list will get accessed (and possibly modified) 5 times!
Hopefully this makes sense. Anyhoo, this is the way I solved that problem and used a common gotcha for good instead of evil. Do you have a better way to solve the problem? Still confused about why the default argument works like a cache or what the difference between mutable and immutable is? Please hit me up at hello@assertnotmagic.com and tell me about it!
Author: Ryan Palo | Tags: python algorithms tricks |Like my stuff? Have questions or feedback for me? Want to mentor me or get my help with something? Get in touch! To stay updated, subscribe via RSS